Pricing [data]points and Predictions: Data Product Pricing Frameworks
TL;DRPricing data products is a different beast from SaaS—value is contextual, and the product layer matters as much as the data.Start by understanding what makes your data valuable (to whom, and why).Layer on product experience: APIs, dashboards, integrations, and support are all pricing levers.Use a mix of usage-based and value-based models; experiment with tiers, access, and outcomes.Don't ignore industry quirks, legal, and compliance—these can make or break your pricing.Treat pricing as a living product: monitor, adapt, and evolve as the market shifts.The future? More AI (all types), more outcome-based pricing, and a premium on privacy and real-time data.
Introduction
Data is often called the new oil, but it's also the new currency—valuable, but only in the right context, and not always fungible (St. Andrews Economist, 2023).
Every data product manager eventually faces the same question: How much is this data actually worth? And how do I price not just the data, but the product built around it?
If you've ever felt like you're making it up as you go along, you're not alone. Pricing data (and data products) is one of the most misunderstood—and most consequential—challenges in the field.
Unlike SaaS, where pricing models are well-trodden and benchmarks abound, data products live in a world of ambiguity. The value of a dataset can change depending on the use case, the user, and even the day of the week. The product layer—APIs, dashboards, analytics—adds another dimension of complexity.
In this post, we'll break down the art (pricing really is art) and science of data product pricing. We'll explore:
- why traditional SaaS pricing models fall short
- how to think about the value of data as an asset
- how to combine asset and product thinking into a practical pricing strategy
Whether you're launching your first data product or rethinking the pricing of an established offering, this guide will help you navigate the unique challenges—and opportunities—of pricing in the data economy. It's a space for me to put down thoughts and share them broadly
Understanding Data Asset Value
Fundamental Principles
Before you can price a data product, you have to understand what gives data its value in the first place. This is where most teams stumble. Data isn't like software code or a physical good—it doesn't have intrinsic value. Its worth is entirely contextual, shaped by who's using it, how, and for what purpose.
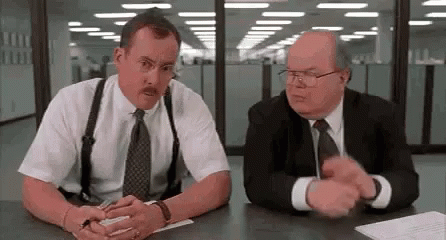
1. Data has no innate value—value derives from utility.
A dataset sitting on a hard drive is just a cost center. It only becomes valuable when it helps someone make a better decision, automate a process, or unlock a new opportunity. For example, a list of retail transactions is worthless to a hospital, but gold to a consumer analytics firm.
2. Value depends on use case and user.
The same dataset can be worth pennies to one customer and millions to another. A hedge fund might pay a premium for satellite imagery of parking lots to predict retail earnings, while a logistics company might see little use for it. Always ask: Who will use this data, and what will they do with it?
3. The additive nature of data.
Data's value often increases when combined with other datasets. A customer list is more valuable when enriched with demographic or behavioral data. This "additive" property means that even seemingly commoditized data can become differentiated through clever integration.
4. Rivalry vs. non-rivalry.
Unlike physical goods, data can be sold to multiple customers without being "used up." But exclusivity can drive up value—if you're the only one with access to a unique dataset, you can command a premium. Decide early: Are you selling exclusivity, or scale?
Value Drivers
What helps establish the "value" and the price you set? Quality + positioning.
Quality Metrics
- Accuracy and completeness: Is the data correct and comprehensive? Inaccurate or missing data erodes trust and value.
- Freshness and timeliness: How up-to-date is the data? For financial trading, even a few seconds' delay can make data worthless.
- Uniqueness and exclusivity: Is this data available elsewhere? Unique or proprietary data is always more valuable.
- Coverage and granularity: Does the data cover the right scope (e.g., all US hospitals) and at the right level of detail (e.g., daily vs. monthly)?
Market Position
- Lifecycle stage: Is your data a new "alpha" source, or has it become a commodity? Early-stage, high-impact data commands higher prices.
- Market maturity: In mature markets, pricing is often driven down by competition. In emerging spaces, you can set the rules.
- Competitive landscape: Are there substitutes? If so, what are their strengths and weaknesses?
- Barriers to entry: How hard is it for others to replicate your data? Proprietary collection methods, regulatory approvals, or unique partnerships can all create defensibility.
Example: When I worked with a healthcare data provider, we found that our claims dataset was only moderately valuable on its own. But when we layered in hospital quality scores and patient satisfaction data, the combined product became a must-have for payers and providers looking to benchmark performance. The value wasn't in the raw data, but in the unique combination and the insights it enabled.
Want more of this in your inbox?
Takeaway:
Before you think about pricing, get brutally honest about what makes your data valuable—and to whom. Map out your value drivers, assess your defensibility, and be clear about whether you're selling a commodity or a crown jewel. This clarity will shape every pricing decision that follows.
Product Layer Pricing
Once you've mapped the value of your data asset, it's time to layer on the product. This is where many data teams get tripped up: they price the data, but forget to price the product experience that surrounds it. In reality, the product layer is often where the most defensible value—and differentiation—lives.
If your data is a commodity, your product experience is your moat. If your data is unique, your product can multiply its value.
Infrastructure Components
Think of this as the "plumbing" that makes your data usable:
- Data processing and storage costs: How much does it cost to clean, process, and store the data? These are your baseline costs, but also a source of value if you can offer faster, more reliable, or more secure delivery than competitors.
- API and delivery mechanisms: Are you providing raw data dumps, or real-time APIs? The latter can command a premium, especially if you offer robust uptime, low latency, and strong documentation.
- Integration capabilities: The easier it is for customers to plug your data into their workflows (via connectors, SDKs, or pre-built integrations), the more you can charge.
- Security and compliance features: In regulated industries, features like audit trails, encryption, and compliance certifications (HIPAA, SOC 2, etc.) are not just table stakes—they're pricing levers.
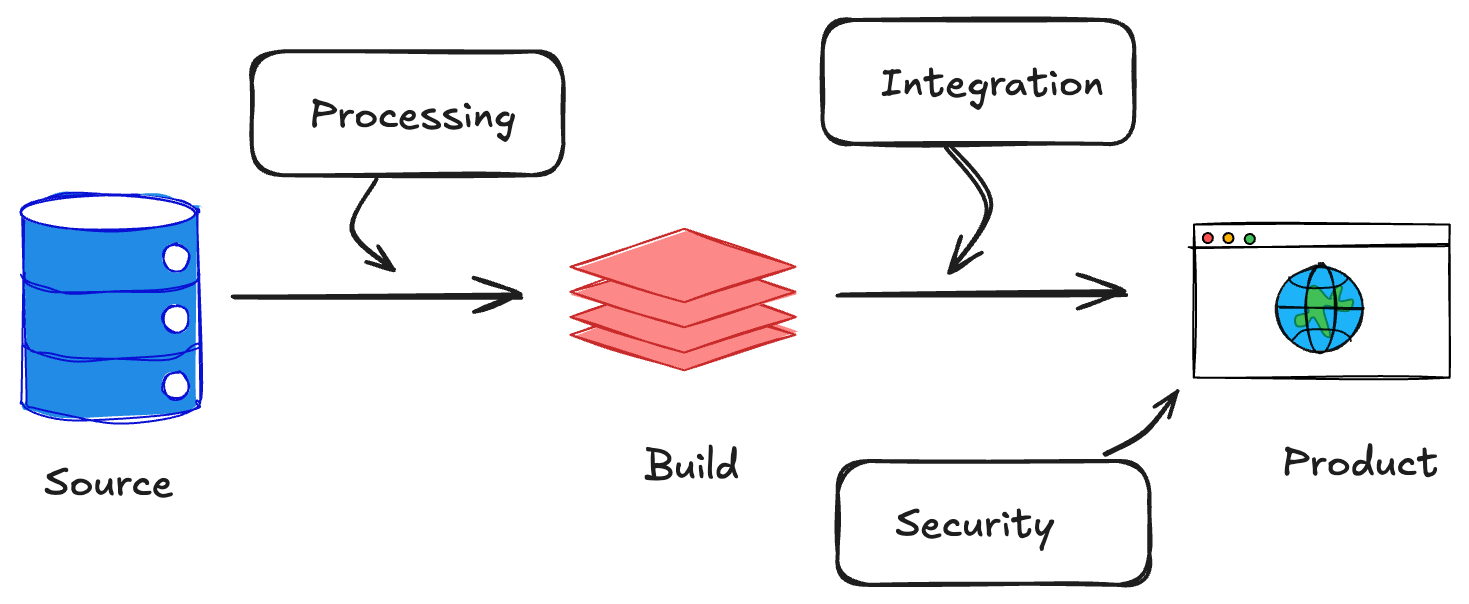
User Experience Elements
This is where you turn data into decisions:
- Interface and visualization: Dashboards, reports, and visualizations make data actionable. A beautiful, intuitive interface can be the difference between a "nice-to-have" and a "must-have."
- Self-service capabilities: Can users explore, filter, and analyze data on their own? Self-service tools reduce support costs and increase perceived value.
- Documentation and support: Great docs and responsive support are often overlooked, but they're critical for adoption—especially for technical buyers.
- Custom features and functionality: Alerts, scheduled reports, or custom analytics can justify higher price points, especially for enterprise customers.
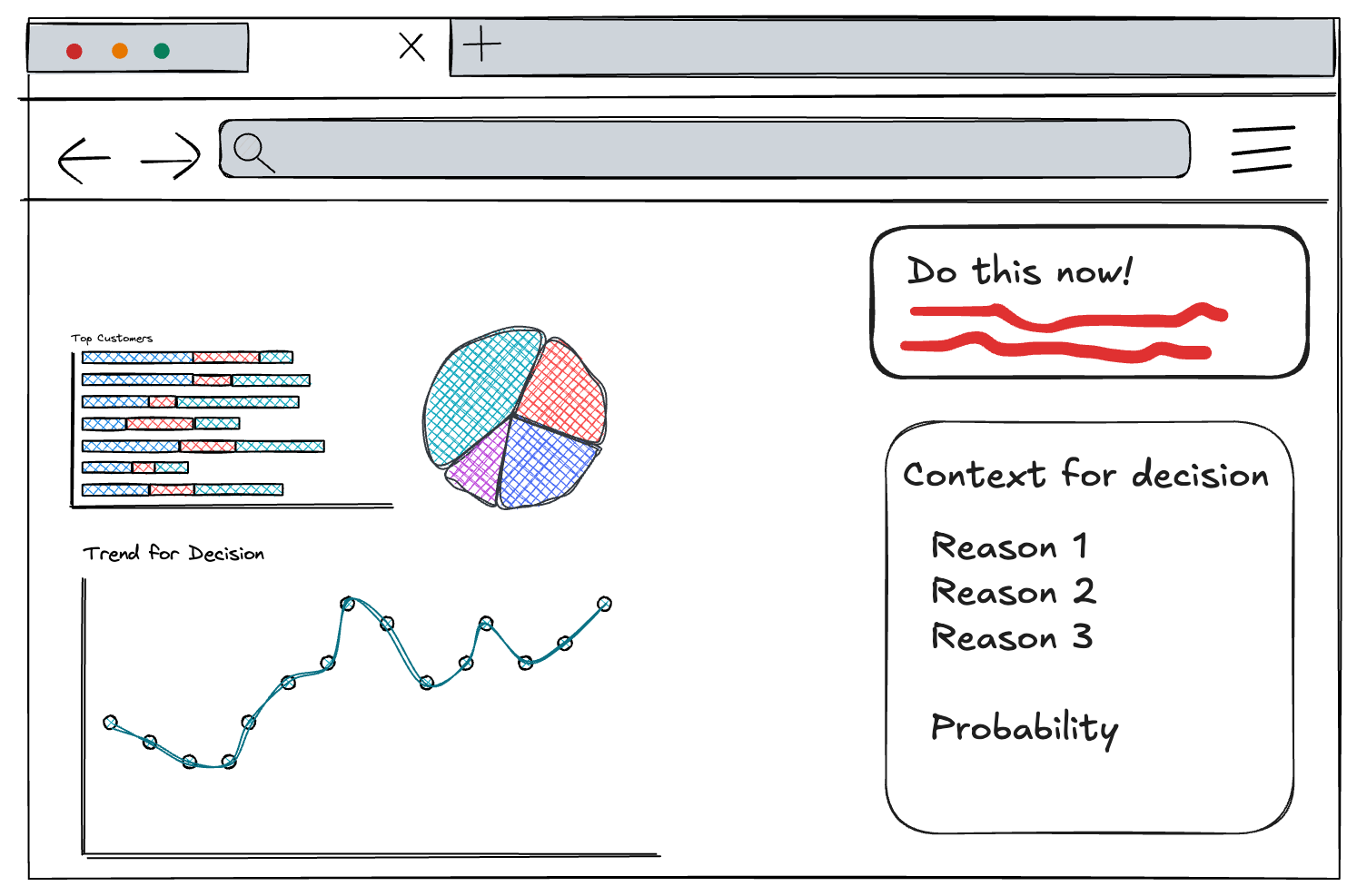
The best data products don't just deliver data—they deliver outcomes and trust. Price for the value your product enables, not just the bytes you ship.
Takeaway:
When pricing your data product, don't stop at the data. Map out every layer of the product experience, from infrastructure to interface. Each layer is a potential pricing lever—and a chance to differentiate in a crowded market.
Combined Pricing Strategies
So, you've mapped your data's value and built a product around it. Now comes the million-dollar question: How do you actually charge for it? The answer is rarely simple, but the best data product pricing strategies blend usage-based and value-based models, with plenty of room for creativity.
There's no one-size-fits-all model - sorry to break it to you. The right pricing strategy depends on your data, your product, your market, and your customers' willingness to pay.

Pricing Models
Usage-Based
Usage-based pricing is intuitive for data products. Customers pay for what they consume—whether that's rows of data, API calls, or number of users. This model aligns cost with value delivered, and scales as your customers grow.
- Volume tiers: Charge based on the amount of data accessed (e.g., 1M rows/month, 10M rows/month, etc.).
- Query-based pricing: Price per query or report generated, common in analytics and BI products.
- API calls: Charge per API request, with discounts for higher volumes.
- User seats: Price by the number of users or seats accessing the product.
Visual suggestion: Table or chart comparing different usage-based pricing tiers, showing how costs scale with usage.
Value-Based
Value-based pricing is about charging for outcomes, not just inputs. This model works best when you can tie your product to a clear ROI or business impact.
- Industry-specific metrics: Price based on metrics that matter to your customer (e.g., dollars saved, revenue generated, risk reduced).
- ROI-based pricing: If your data product helps a customer save $1M, charging $100K is a no-brainer.
- Outcome-based models: Charge only when a certain result is achieved (e.g., successful fraud detection, leads generated).
- Risk-sharing arrangements: Offer performance guarantees or share in upside to align incentives.
Value-based pricing requires deep customer understanding and trust. It's harder to implement, but can unlock much higher price points.
Pricing Levers
These are the dials you can turn to fine-tune your pricing and segment your market:
- Access levels: Raw data vs. processed data, or basic vs. premium features.
- Update frequency: Real-time updates command a premium over daily or weekly refreshes.
- Historical depth: More history = higher price.
- Geographic coverage: Global data costs more than regional or local.
- Usage rights and restrictions: Exclusive access, redistribution rights, or white-labeling can all justify higher prices.
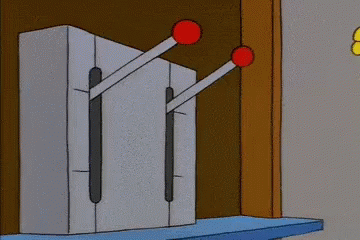
Framework: Building Your Pricing Model
- Start with your value drivers: What do your best customers care about most?
- Choose your base model: Usage-based, value-based, or a hybrid.
- Layer on pricing levers: Create tiers or packages that match different customer needs.
- Test and iterate: Pricing is never "set and forget." Talk to customers, run experiments, and adjust as you learn.
Takeaway:
The best data product pricing strategies are flexible, transparent, and aligned with customer value. Don't be afraid to experiment—and remember, your pricing model is a product in itself.
Implementation Framework
You've mapped your value, built your product, and chosen a pricing model. Now it's time to put it all together in a way that's both rigorous and adaptable. The best pricing frameworks are grounded in reality—your costs, your market, and your customers' needs.
Pricing is a process, not a one-time event. The best teams revisit and refine their pricing as their product and market evolve.
Assessment Phase
Internal Analysis
- Cost structure: Start by understanding your true costs—data acquisition, processing, storage, delivery, support, and compliance. Don't forget hidden costs like customer onboarding or custom integrations.
- Marginal costs: For most data products, the cost to serve one more customer is low, but not zero. Know your margins so you can price profitably.
- Scalability factors: Can your infrastructure handle 10x more customers? If not, factor in the cost of scaling.
- Operational constraints: Are there limits to how much data you can deliver, or how quickly you can onboard new clients?
Visual suggestion: Cost breakdown chart or waterfall diagram showing how costs accumulate from raw data to delivered product.
Market Analysis
- Customer segmentation: Not all customers are created equal. Segment by industry, company size, use case, or willingness to pay.
- Competitive positioning: Map your competitors' offerings and pricing. Where can you differentiate? Where are you at risk of commoditization?
- Market size and potential: Is your market growing, shrinking, or saturated? This will shape your pricing ambition.
- Industry-specific factors: Regulatory requirements, procurement cycles, and industry norms can all impact pricing.
Talk to real customers early and often. Pricing is as much about perception as it is about math.
Pricing Structure Design
Base Components
- Core offering definition: What's included in your base package? Be clear about what's "standard" and what's extra.
- Minimum viable product: Don't overbuild. Start with the smallest set of features that delivers real value.
- Essential features: Identify the must-haves for your target segment. Everything else can be an add-on or upsell.
Premium Elements
- Advanced capabilities: Machine learning, predictive analytics, or custom reporting can justify higher tiers.
- Enhanced service levels: Faster SLAs, dedicated support, or custom onboarding are all premium levers.
- Custom solutions: For enterprise clients, be ready to price bespoke integrations or data feeds.
- Professional services: Training, consulting, or data science services can be valuable add-ons.
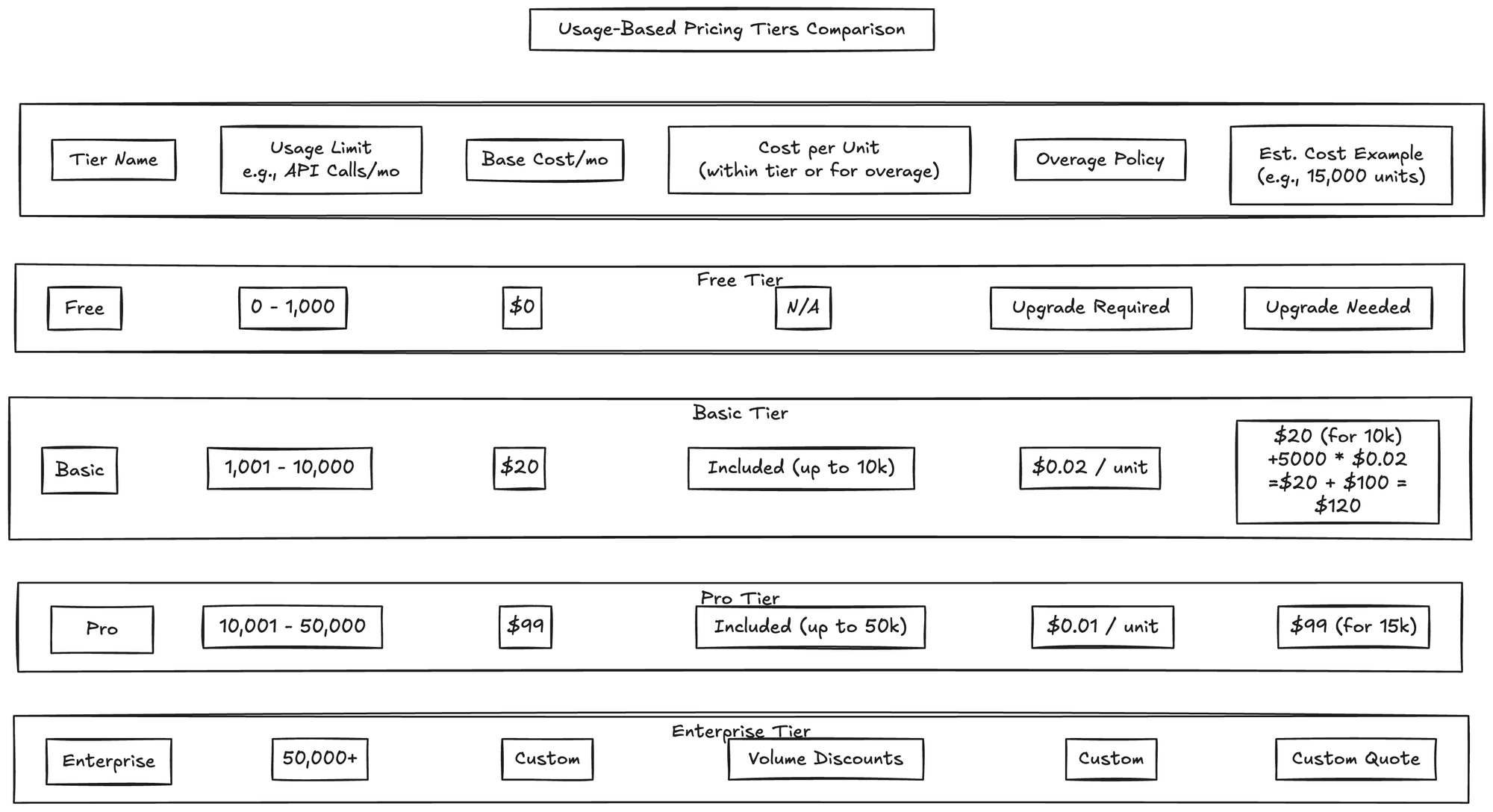
Example:
A B2B data platform might offer a "Starter" tier with basic data access and self-service analytics, a "Professional" tier with advanced integrations and premium support, and an "Enterprise" tier with custom data feeds and dedicated account management. Each tier is priced to match the value delivered—and the cost to serve.
Takeaway:
A robust implementation framework grounds your pricing in reality and gives you the flexibility to adapt as you learn. Don't be afraid to start simple and add complexity as your product and market mature.
Special Considerations
Even the best pricing model can be derailed by industry quirks, legal landmines, or compliance hurdles. Data products live at the intersection of technology, regulation, and business—and each industry brings its own set of challenges.
Ignore industry and legal context at your peril. The right pricing in the wrong regulatory environment can kill a deal—or your whole business.
Industry-Specific Factors
- Financial services: Data freshness and accuracy are paramount. Regulatory requirements (e.g., SEC, FINRA) can dictate how data is delivered and priced. High-value, low-latency data often commands a premium, but must be auditable and secure.
- Healthcare: HIPAA and other privacy laws shape what data can be sold, to whom, and how it must be protected. De-identification, consent, and audit trails are not just features—they're requirements. Pricing must reflect the cost and risk of compliance.
- Marketing/AdTech: Privacy regulations (GDPR, CCPA) are tightening. Data provenance, consent management, and opt-out mechanisms are now table stakes. Expect more scrutiny on how data is sourced and used.
- AI/ML training data: The value of training data is skyrocketing, but so are concerns about bias, copyright, and ethical use. Pricing must account for exclusivity, labeling quality, and downstream liability.
Visual suggestion: Matrix or infographic showing how pricing levers and compliance requirements vary by industry (e.g., healthcare vs. finance vs. AdTech).
Legal and Compliance
- Data ownership: Who owns the data you're selling? If it's aggregated from third parties, do you have the rights to resell it? Clear contracts and provenance are essential.
- Usage rights: Define exactly how customers can use your data—internal analysis, redistribution, commercial resale, etc. More rights = higher price (and higher risk).
- Privacy regulations: Stay current on global privacy laws. Non-compliance can mean massive fines and reputational damage.
- Industry compliance: Certifications (SOC 2, ISO 27001, HITRUST) can be a differentiator—and a pricing lever—in regulated markets.
Legal and compliance costs aren't just overhead—they're part of your value proposition. Customers will pay more for data they can trust.
Takeaway:
Build compliance and industry context into your pricing from day one. It's easier (and cheaper) to get it right up front than to retrofit your product or pricing later.
In a world of infinite, AI-generated content, the ability to prove your data's origin, quality, and curation will command a premium. Trust chains and provenance are the new differentiators (Pivotal, 2023).
Optimization and Evolution
Pricing isn't a "set it and forget it" exercise. The best data product teams treat pricing as a living system—constantly monitored, tested, and refined as the market, product, and customer needs evolve.
Your first pricing model will be wrong. The winners are those who learn and adapt the fastest.
Monitoring and Metrics
- Usage patterns: Track how customers actually use your product. Are they hitting usage caps? Abandoning after onboarding? These signals can reveal both pricing friction and upsell opportunities.
- Customer feedback: Regularly ask customers about perceived value, pain points, and what would make them pay more (or less).
- Market changes: Keep an eye on competitors, new entrants, and shifting industry standards. What was premium last year may be table stakes today.
- Cost evolution: As your infrastructure scales, your cost structure will change. Revisit your margins and adjust pricing as needed.
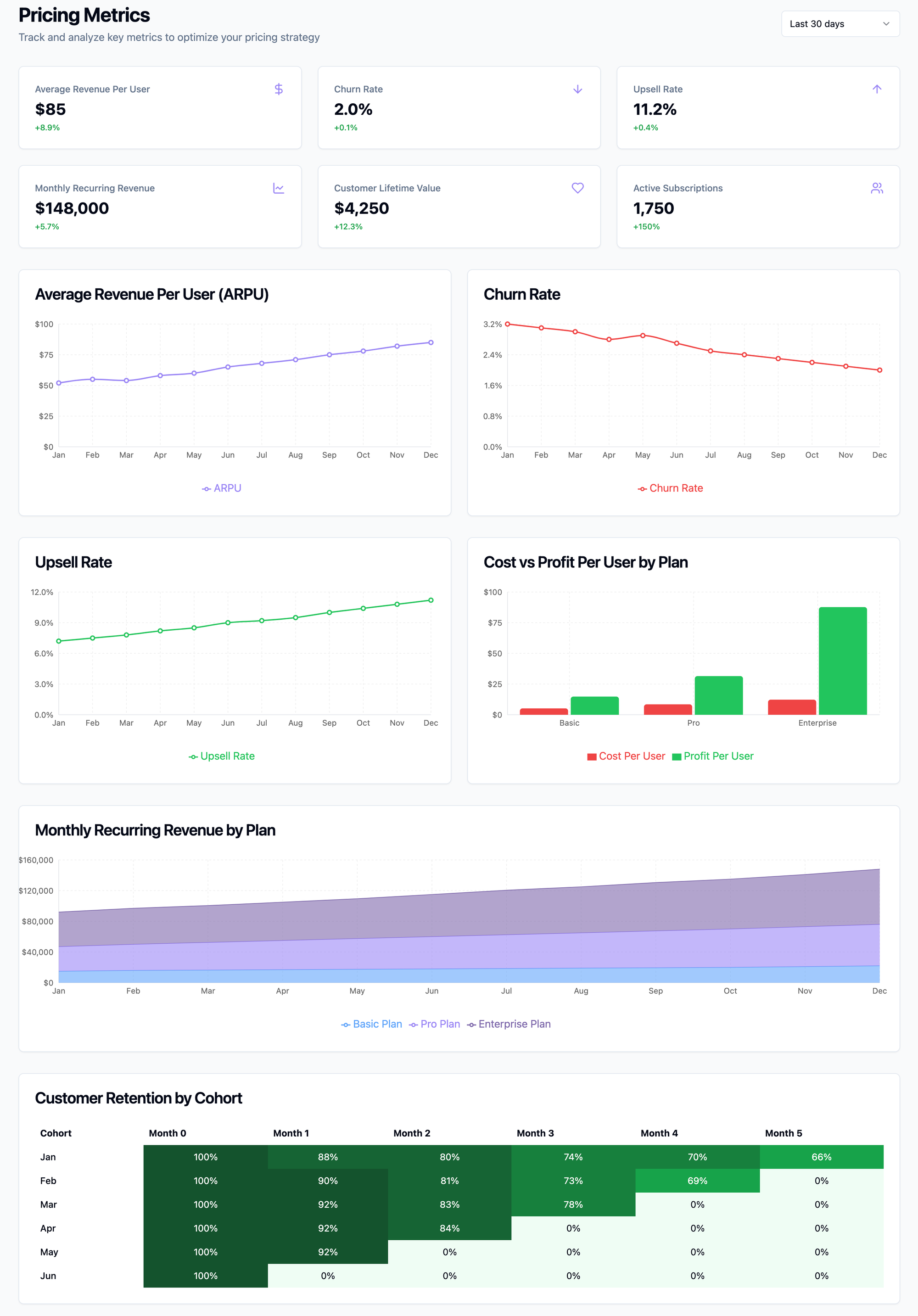
Adaptation Strategies
- Version upgrades: Use new features or data sources as opportunities to revisit pricing. Don't be afraid to grandfather existing customers while raising prices for new ones.
- Market expansion: As you move into new segments or geographies, test different pricing models to find the right fit.
- Feature additions: Launching a major new capability? Consider a new tier or add-on rather than bundling it into your base price.
- Pricing adjustments: Don't wait for a crisis to adjust pricing. Small, regular tweaks are less risky than big, infrequent overhauls.
Communicate pricing changes transparently and early. Customers are more forgiving when they understand the "why."
Takeaway:
Treat your pricing like a product—iterate, test, and improve. The most successful data product companies are relentless about learning from the market and evolving their pricing to match.
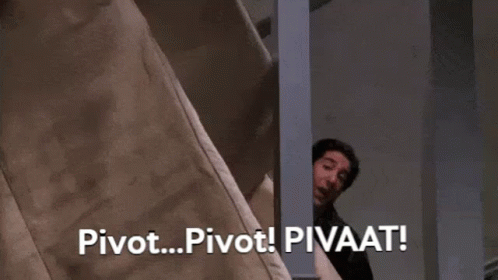
Specific Pricing Frameworks by Data Product Type
No two data products are priced the same. The right framework depends on your data's nature, your customers, and your market. Here's how to approach pricing for the most common data product types, with examples and callouts for what makes each unique.
1. Market Data Products
Market data (e.g., financial, economic, or industry feeds) is all about speed, accuracy, and coverage. Customers pay for freshness and reliability.
- Pricing approach: Tiered by latency (real-time, delayed), history depth, and coverage. Premium for real-time, global, or exclusive access.
- Unique considerations: Regulatory requirements, auditability, and SLAs are often non-negotiable.
- Example: A stock market data provider might offer delayed data for free, real-time for a fee, and exclusive pre-market feeds at a premium.
2. Alternative Data Products
Alternative data (e.g., satellite imagery, web scraping, IoT signals) is prized for its uniqueness and alpha potential.
- Pricing approach: Priced by uniqueness, exclusivity, and integration complexity. Custom deals are common.
- Unique considerations: Data provenance, legal rights, and integration support are key differentiators.
- Example: A hedge fund pays a premium for exclusive access to a new geolocation dataset that predicts retail foot traffic.
The rarer the data, the more you can charge—especially if you offer exclusivity.
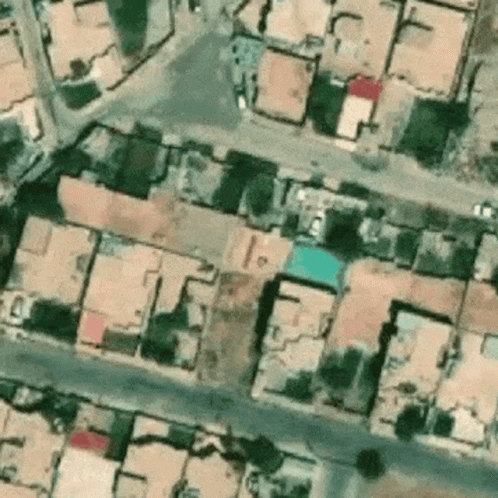
3. B2B Data Intelligence Products
These products (e.g., company info, contact data, industry insights) are all about breadth, accuracy, and enrichment.
- Pricing approach: Tiered by records accessed, enrichment level, and user seats. API access and export limits are common levers.
- Unique considerations: Data freshness, compliance (GDPR), and enrichment features drive value.
- Example: A sales intelligence platform charges by the number of contacts accessed and the depth of company profiles.
4. Consumer Data Products
Consumer data (e.g., demographics, behavior, transactions) is highly regulated and privacy-sensitive.
- Pricing approach: Segmented by audience size, data freshness, and segmentation complexity. Premium for custom segments or analytics.
- Unique considerations: Privacy compliance (GDPR, CCPA), consent management, and opt-out mechanisms are essential.
- Example: A marketing platform charges more for real-time behavioral data and custom audience segments.
5. AI Training Data Products
Training data for AI/ML models is all about quality, labeling, and exclusivity.
- Pricing approach: Priced by data volume, labeling quality, and exclusivity. Custom collections and co-creation deals are common.
- Unique considerations: Bias, copyright, and downstream liability must be addressed in contracts and pricing.
- Example: An AI startup pays a premium for a large, expertly labeled medical image dataset, with exclusivity for a year.
6. IoT/Sensor Data Products
IoT data (e.g., sensor feeds, telemetry) is valued for real-time access and integration ease.
- Pricing approach: Tiered by number of sensors, data frequency, and storage duration. Platform access and analytics tools are upsell levers.
- Unique considerations: Integration APIs, uptime guarantees, and data retention policies matter.
- Example: A logistics company pays for real-time fleet tracking, with higher tiers for historical analytics and API integrations.
7. Analytics Data Products
Analytics products (e.g., dashboards, reporting tools) turn raw data into insights and decisions.
- Pricing approach: Tiered by data volume, processing complexity, user seats, and export capabilities. Freemium models are common for self-service tools.
- Unique considerations: Custom reporting, white-labeling, and advanced analytics can justify premium tiers.
- Example: A BI platform offers a free tier for basic analytics, with paid plans for advanced features and higher data limits.
Implementation Guidelines
- Start with the simplest pricing model that captures value for your segment.
- Consider hybrid models for different customer types (e.g., usage-based for SMBs, custom contracts for enterprise).
- Build in scalability and clear upgrade paths.
- Plan for customization requests—these are often your highest-margin deals.
Common Patterns
- Freemium works best for high-volume, self-service products.
- Enterprise pricing is needed for high-touch, complex solutions.
- Usage-based pricing aligns well with customer value and growth.
- Tiered access helps segment the market and drive upsell.
- Custom pricing is often required for unique or high-value datasets.
Takeaway:
There's no universal playbook, but these frameworks will help you match your pricing to your product's unique strengths and your customers' real needs. Use visuals, clear tiering, and transparent value drivers to make your pricing easy to understand—and easy to buy.
Future Trends
The only thing certain about data product pricing? It's going to change—fast. New tech, new rules, and new customer demands are rewriting the playbook every year. Here's what to keep on your radar as you build for tomorrow.
Change is the only constant. The best teams stay curious, flexible, and close to their customers.
AI/ML: The New Data Gold Rush
- Training data is the new oil. As AI explodes, everyone wants high-quality, well-labeled data. Expect bidding wars, exclusive deals, and new marketplaces for the best sources.
- Synthetic data is coming. Tools that generate "fake but useful" data will shake up pricing. Scarcity and exclusivity will mean something different.
- Performance-based pricing. Some teams will charge not for the data itself, but for how well your models perform with it—think "pay-per-accuracy" or "pay-per-insight."
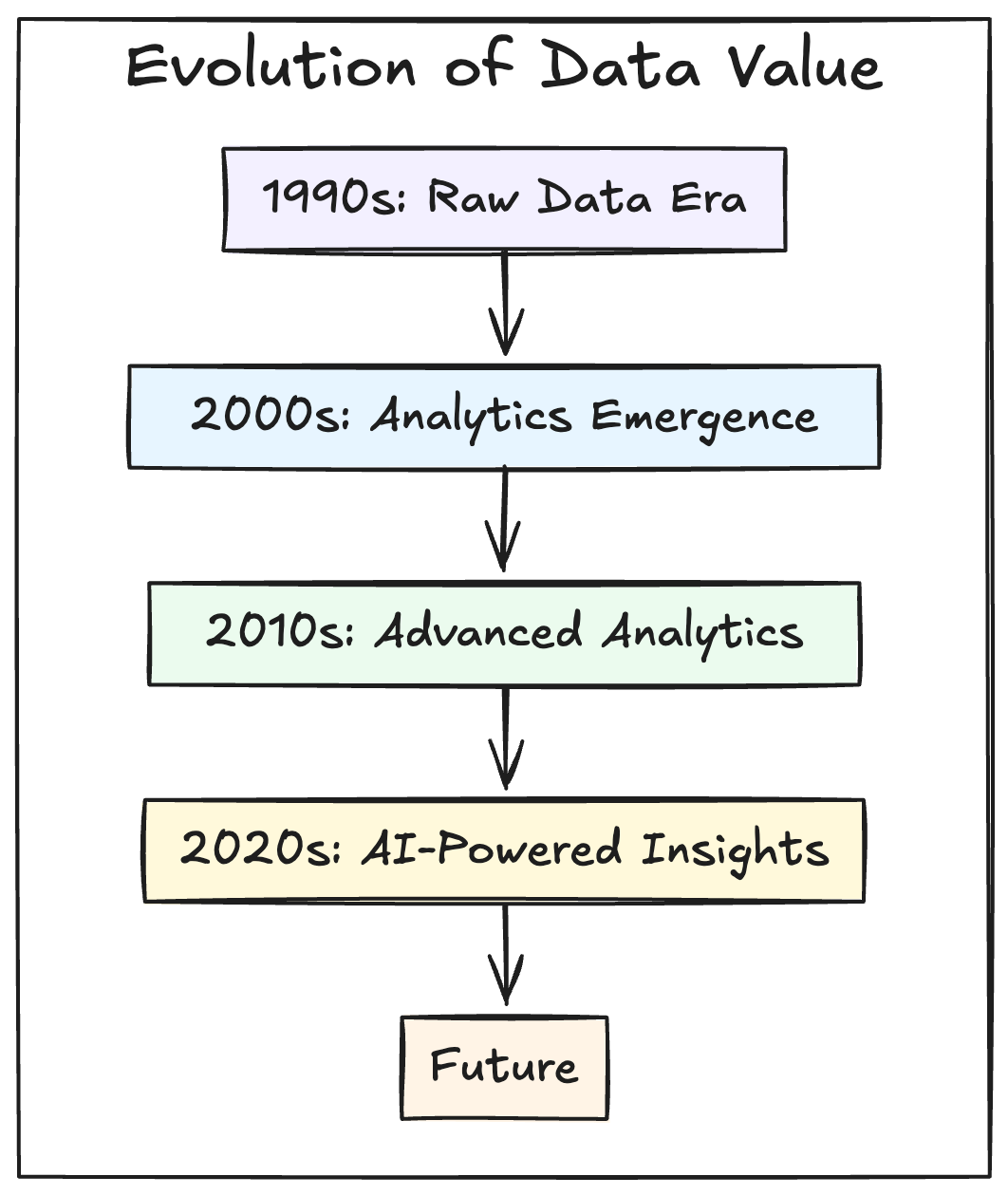
New Pricing Models on the Rise
- Pay for results, not just data. More vendors will tie pricing to business outcomes. "Pay for performance" and risk-sharing models are on the rise.
- Microtransactions everywhere. As APIs and self-serve tools grow, expect more "pay as you go"—per API call, per insight, per user.
- Data-as-a-service (DaaS). Bundled subscriptions that include data, infrastructure, and analytics will keep growing, especially for smaller companies.
The Market Is Moving—Are You?
- Privacy-first wins. With new privacy laws, products that make compliance and transparency easy will stand out—and can charge more.
- Real-time is the new normal. As tech improves, customers will expect instant data and insights. Speed will be a premium feature.
- Mix-and-match data products. Modular, composable data products (think "data mesh") will let customers build what they need—and pay for only what they use.
Takeaway:
The future belongs to teams who treat pricing as a living product. Stay close to your customers, watch the market, and don't be afraid to reinvent your approach as the world changes.
As AI makes compute cheap and abundant, the real scarcity—and value—shifts to unique, high-quality data. The next gold rush is for "golden data" that can power and differentiate AI models (Pivotal, 2023).
References
- How To Price A Data Asset by Abraham Thomas
- Data in the Age of AI by Abraham Thomas
- Data Product Costs as a First Step to Data Product Value by Yuliia Tkachova
Further Reading & Academic References
- The evolution of data pricing: From economics to computational intelligence
- A Survey on Data Pricing: From Economics to Computation
- Data pricing in the digital economy: A systematic review
- 6 Tips for Setting Your Product Price
- Data pricing in data markets: A review
- Data: The New Form of Currency
- Data pricing and privacy: A review