Positioning and Messaging Framework for Data Product Managers
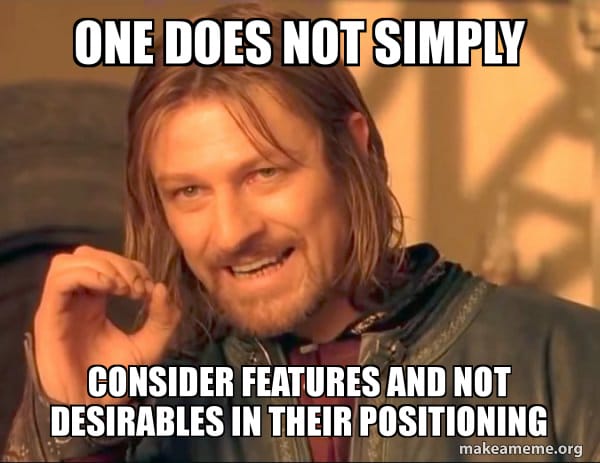
Why Data Product Managers Need Their Own Positioning Framework
"Positioning defines how your product is a leader at delivering something that a well-defined set of customers cares a lot about." - April Dunford
When I started my journey in data product management, I faced a reality that many technical folks encounter: we're really good at building things, but sometimes terrible at explaining why anyone should care.
I once watched a brilliant data science team build an incredible patient flow optimization tool for a hospital system. It used cutting-edge algorithms, beautiful visualizations, and could accurately predict staffing needs 48 hours in advance with 94% accuracy.
Nobody used it.
Why? The positioning was all wrong. The team pitched it as "a machine learning model that predicts staffing requirements using time-series forecasting of patient flow metrics." The hospital executives needed "a cost-saving tool that reduces overtime expenses while maintaining quality care standards."
Same product. Completely different positioning.
If you're a data product manager (or leading a team that builds data products), you face unique positioning challenges that traditional software PMs don't encounter. The frameworks they use simply don't account for the complexities of communicating data value.
Positioning as Context-Setting
Positioning expert April Dunford describes positioning as "context-setting for products" – like the opening scene of a movie that orients viewers to what they're about to experience. Note: April has an incredible quickstart guide for positioning available here. When you position your data product, you're setting off powerful assumptions about:
- Who your product competes with
- What features your product should have
- Who the product is intended for
- What the product should cost
Good positioning creates assumptions that are true. Bad positioning creates assumptions you'll spend months trying to undo.
For data products specifically, this is critical. Say "analytics dashboard" and stakeholders immediately assume certain things. Say "operational risk prediction system" and they assume something completely different – even if both descriptions refer to the same product.
Why Data Product Positioning Is Different (And Harder)
The uncomfortable truth is that most data products fail at positioning for some predictable reasons:
- The curse of knowledge - When you've spent months cleaning datasets and fine-tuning algorithms, it's hard to remember what it's like not to understand the technical achievement.
- Multiple stakeholder perspectives - A CFO, a frontline manager, and a data analyst will all see your product through different lenses, requiring different positioning.
- Invisible competitors - You're not just competing with other data products; you're competing with Excel, gut feelings, and the status quo.
- The capability trap - Data teams love to talk about what their product can do rather than what problems it solves.
I've seen brilliant data products die quiet deaths because they couldn't cross the positioning chasm. The good news? A structured framework can help.
A Customer-Centric Approach to Positioning
Traditional positioning exercises often start with a "positioning statement" – a fill-in-the-blank template that assumes you already know your category, competitors, value proposition, and target customers.
But as April Dunford points out in her work on positioning, this approach is backward. You can't create effective positioning by starting with the statement. You need to work through a methodical process to discover your best positioning.
For data products, this process becomes even more critical because of the inherent complexity and the technical-business translation challenge.
The Five Components of Positioning for Data Products
Dunford's framework breaks positioning into five key components that build on each other:
- Competitive Alternatives - What would customers do if your data product didn't exist?
- Unique Attributes - What do you have that alternatives don't?
- Value for Customers - What value do those unique attributes enable?
- Target Customers - Who cares a lot about that value?
- Market Category - What context makes your value obvious to those customers?
Let's apply this specifically to data products:
1. Identify True Competitive Alternatives
For data products, the most dangerous mistake is defining your competitors as other data products. In reality, your true competition is often:
- Manual Excel analysis
- Weekly status meetings
- Gut-feeling decision making
- Email-based reporting
- Outsourced analytics services
- Doing nothing at all
In healthcare settings, I've seen data products position themselves against "traditional BI tools" when they should have positioned against "the monthly manual chart reviews that PA's (physician assistants) hate doing."
With a Chamber of Commerce project, board members were using quarterly PDF reports, while business leaders were cobbling together information from multiple government websites. Two completely different alternatives that required different positioning strategies.
2. Highlight Genuinely Unique Attributes
Once you understand what you're replacing, identify what your data product has that those alternatives don't. For data products, differentiators often include:
- Real-time data processing capabilities
- Cross-system data integration
- Advanced anomaly detection
- Automated pattern recognition
- Predictive modeling
- Contextual recommendations
The key here is to focus on attributes that are actually unique compared to the true alternatives – not just features that sound impressive.
3. Translate Attributes to Value
This is where data product teams typically struggle most. Technical capabilities must be translated into value that business stakeholders understand. Instead of "faster" and "more accurate," frame advantages in terms of:
- Operational value: Saves time, reduces errors, simplifies workflows
- Strategic value: Enables new capabilities, uncovers hidden opportunities, reduces risk
- Transformational value: Changes fundamental business models, creates new revenue streams
When we repositioned our healthcare revenue analytics tool from "more accurate prediction" to "prevention of $3.2M in denied claims annually," suddenly the CFO started showing up to our demos.
4. Identify Who Cares Most About Your Value
Not all potential users will value your data product equally. Identify segments that care deeply about the specific value you provide.
When building a patient experience data product, we learned that Chief Patient Experience Officers needed radically different information than CEOs or nursing managers:
- For CEOs: Positioned as an executive decision support tool connecting patient satisfaction to financial outcomes and competitive positioning
- For Nursing Managers: Positioned as an operational improvement tool identifying specific service recovery opportunities
- For Chief Experience Officers: Positioned as a comprehensive analytics platform supporting detailed program development
Each required different language, different metrics, and even different UX patterns.
Remember: Data products that try to serve everyone equally end up serving no one effectively.
5. Choose the Right Market Category
Your market category creates the context for how people understand your product. For data products, the right market category isn't always obvious.
When working with a Chamber of Commerce on their economic development data product, we initially positioned it as "an interactive data visualization tool for economic indicators." After repositioning it as "a business expansion decision support system that quantifies market opportunities," engagement with local business leaders increased dramatically.
Be cautious about creating entirely new categories. As Dunford notes, while it can be tempting to create a new market category (like "Decision Intelligence Platform" or "Operational Analytics Hub"), most successful tech companies position in existing markets first before stretching boundaries.
Applying This Framework: Before and After
Here's the before/after transformation of a positioning statement for a healthcare data product:
Before:
"An advanced analytics platform leveraging machine learning to provide multi-dimensional insights into patient satisfaction across service lines."
After:
"A decision support tool for hospital executives that transforms patient survey data into actionable improvement plans, replacing manual report analysis with automated priority identification that reduces time-to-improvement by 60%."
The second version answers all four key questions clearly:
- What is it? (A decision support tool)
- Who is it for? (Hospital executives)
- What does it replace? (Manual report analysis)
- Why is it better? (Reduces time-to-improvement by 60%)
The SLC Framework for Iterative Positioning
I'm a big believer in the Simple, Lovable, Complete (SLC) framework for product development. Your positioning should follow the same principle:
- Simple: One clear value proposition that anyone can understand
- Lovable: Addresses a painful problem in a way that resonates emotionally
- Complete: Covers what it is, who it's for, what it replaces, and why it's better
Common Data Product Positioning Pitfalls
In my years consulting with data teams, I've seen the same mistakes again and again:
- The jargon trap: Using terms like "machine learning," "predictive analytics," or "AI-powered" without explaining the actual benefit to users.
- The capability focus: Listing all the things your product can do without connecting them to user problems or goals.
- The all-things-to-all-people problem: Trying to serve everyone from data scientists to executives with the same positioning.
- The missing "so what" factor: Failing to make explicit why users should care about the insights your product provides.
- The phantom competitor trap: Positioning against competitors that your customers don't actually consider, rather than positioning against what they'd genuinely do without your product.
A simple test: If you read your positioning statement to a non-technical stakeholder, would they immediately understand what problem you solve and why it matters to them?
Apply This Framework Today
Want to improve your data product's positioning right now? Here's a quick-start template:
[Product Name] is a [product category] for [specific target audience] that [key problem it solves] by [how it works] unlike [main alternative], which [key limitation of alternative].
For a healthcare claims analytics product, that might be:
ClaimSight is a denial prevention tool for hospital revenue cycle managers that reduces claim rejections by 32% by predicting likely denials before submission, unlike traditional claims scrubbing software, which only checks for technical errors after claims are prepared.
When working with your team, ask these questions:
- What would our customers do if our solution didn't exist?
- What unique capabilities do we have compared to those alternatives?
- What value do those capabilities create for customers?
- Which customer segments care most about that value?
- What market category makes our value obvious to those customers?
The Bottom Line
As April Dunford puts it, positioning is not just marketing fluff – it's the bedrock of your go-to-market strategy. For data products specifically, it can make the difference between a technically impressive solution that nobody uses and a business-critical tool that transforms organizations.
How you position your product determines what features you prioritize, how you design the interface, and which stakeholders you engage. Great data products speak human, not just machine. They translate complex analytics into language that resonates with real people trying to solve real problems.
The next time you're building something brilliant with data, remember: your sophisticated algorithms deserve equally sophisticated positioning.
Check out more of April's work at aprildunford.com