Beyond the Product Trio - Why Data Products Need a Squad
Data products need more than a trio.
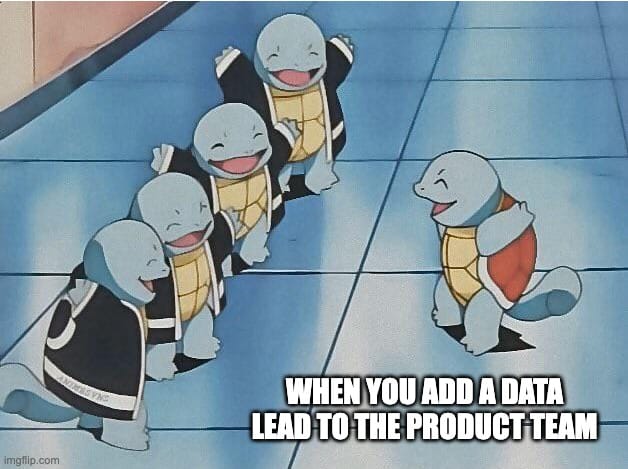
Growth is driven by compounding, which always takes time. Destruction is driven by single points of failure, which can happen in seconds, and loss of confidence, which can happen in an instant. - Morgan Housel, Psychology of Money
The Team Behind Market Profiler
In my second year at Revive, I was tasked with turning a one-off healthcare market analysis project into a scalable product offering. We'd delivered a bespoke market analysis to a regional health system that had generated significant strategic impact, and leadership wanted to create a repeatable data product that could be sold to multiple clients.
Armed with a PowerPoint, Tableau workbook, and an analyst who'd crafted some impressive Alteryx/Python data pipelines, we gathered to map a way forward
The kickoff meeting included our typical product development cast: me as Product Manager, a Tech Lead responsible for implementation, and a Design Lead to craft the user experience. This tried-and-true "Product Trio" had successfully launched numerous healthcare marketing campaigns and products before.
But something wasn't clicking.
"How confident are we in the predictive model's accuracy across different market types?" asked the Tech Lead.
"What about demographic blind spots? Rural markets have different data coverage than urban ones," noted the Designer.
"And the ethical implications of giving health systems competitor intelligence? What are the elements missing between the claims, EHR, and other data sources we are considering? Are we sure about this?" I wondered aloud.
While I had the coverage and experience previously, I needed support and deep data expertise. Not just coding skills or database knowledge, but someone who who had seen healthcare data's nuances, limitations, and ethical boundaries.
The next week, I added a Data Lead to our core team - a data engineer. We moved faster and with more certainty from then on.
This was top of mind when building the next product and the team supporting it. We needed more than the standard trio of leads - we needed a squad.
The Traditional Product Trio
For years, the product development world has operated with a well-established core team structure known as the Product Trio:
- Product Manager: The voice of the market, business, and strategic direction
- Tech Lead: The technical feasibility and implementation expert
- Design Lead: The user experience and interface architect
As Teresa Torres describes it, "A product trio is typically comprised of a product manager, a designer, and a software engineer. These are the three roles that—at a minimum—are required to create good digital products."
This triad works beautifully for traditional software products. The PM understands what to build, the Tech Lead knows how to build it, and the Design Lead ensures it's intuitive and enjoyable to use.
For a typical SaaS product, this structure covers the essential disciplines needed to take a product from concept to market. Technical feasibility questions focus on software engineering challenges: Can we build this feature? How long will it take? Will it scale?
But data products are different beasts entirely.
Enter the Data Product Squad
Data products—whether dashboards, predictive models, recommendation engines, or AI-powered tools—have unique complexities that the traditional trio isn't equipped to fully address.
Enter the Data Product Squad:
- S: Strategic
- Q: Quality-focused
- U: User-centered
- A: Analytical
- D: Data-driven
At its core, the Squad consists of four essential leaders:
- Product Manager: Still the market and business expert, but with awareness of data's unique challenges
- Tech Lead: Focused on system architecture, API design, and overall implementation
- Design Lead: Creating interfaces that make complex data intuitive and actionable
- Data Lead: The data science, engineering, and ethical governance expert
The Data Lead isn't an optional add-on or a nice-to-have consultant. They're an essential fourth pillar in data product development—equal in importance to the other three roles.
The Five Risks of Data Products
Why is this fourth role so critical? Because data products face a risk profile fundamentally different from traditional software.
In his classic work on product risk, Marty Cagan of Silicon Valley Product Group discusses the "Four Big Risks" that all product teams must address:
- Value risk: Will customers buy it or users choose to use it?
- Usability risk: Can users figure out how to use it?
- Feasibility risk: Can our engineers build what we need with the time, skills, and technology we have?
- Business viability risk: Does this solution work for the various aspects of our business?
For traditional products, the Product Trio maps cleanly to these risks:
- The Product Manager addresses value and business viability risks
- The Designer handles usability risk
- The Tech Lead tackles feasibility risk
But data products introduce a fifth critical risk:
5. Ethical Data Risk
This encompasses:
- Accountability for algorithmic decisions
- Representativeness of data
- Fairness across populations
- Transparency and explainability
- Data privacy and governance
- Long-term impact and unintended consequences
This fifth risk doesn't map neatly to the traditional trio. While product managers might understand the business implications, designers might consider the user experience impact, and engineers might recognize some technical limitations, none are typically equipped to fully own this critical risk dimension.
That's where the Data Lead becomes essential.
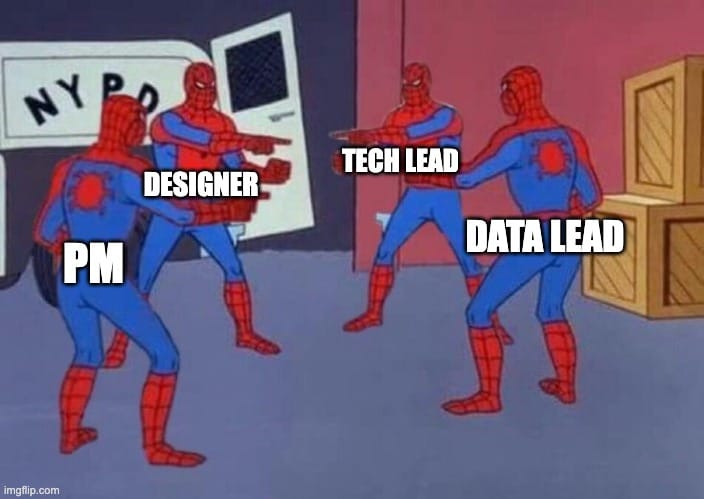
Technical Feasibility Risk (Reimagined)
Even the nature of feasibility risk is different for data products:
In traditional software development, technical feasibility usually centers on engineering challenges: Can we build this feature? How much will it cost? How long will it take?
For data products, feasibility questions are more complex:
- Do we have enough high-quality data to train this model?
- Can we get acceptable accuracy across all key demographics?
- Is real-time prediction possible given our infrastructure?
- How do we handle data drift over time?
These questions require deep expertise in data science, data engineering, and the specific domain's data landscape. A traditional Tech Lead, while brilliant in software engineering, often lacks this specialized knowledge.
Ethical Risk (Expanded)
The ethical dimension permeates data products, especially those using AI/ML:
- Are we accidentally encoding bias in our algorithms?
- Are our recommendations creating harmful incentives?
- Do our visualizations inadvertently mislead users?
- Are we properly protecting sensitive data while still deriving value?
- Can we explain how our model makes decisions?
- Do we have proper measures to monitor, detect, and mitigate failures?
These aren't just hypothetical concerns—they're existential risks for data products. One ethical misstep can destroy trust permanently.
As the quote at the beginning reminds us: growth compounds slowly, but destruction can happen in an instant. For data products, that destruction often stems from ethical oversights that a traditional product team might miss.
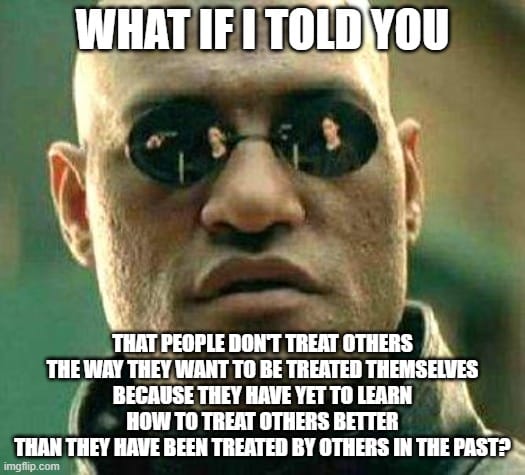
Market Profiler: The Squad in Action
Returning to our Market Profiler example, adding a Data Lead transformed our approach in several crucial ways:
First, our Data Lead immediately identified critical limitations in our demographic data sources. Rural zip codes had significantly less reliable commercial data than urban ones, creating a blind spot that could lead healthcare clients to underinvest in underserved communities. We hadn't fully recognized this issue in our one-off project, but scaling it as a product would have magnified the problem.
Second, he challenged our machine learning approach for predicting service-line growth opportunities. Our initial model used classic propensity scoring, but she demonstrated how this could inadvertently prioritize wealthy, well-insured patients over those with greater needs. We pivoted to a more balanced methodology that considered both commercial opportunity and community health impact.
Finally, he designed a data governance framework that allowed us to provide competitive intelligence without crossing ethical boundaries around protected health information. This included specialized aggregation techniques that prevented reverse-engineering of sensitive metrics.
The result? Market Profiler evolved from an interesting analytics project into a responsible, ethical data product that hospitals could confidently use for strategic planning. Within a year, we had signed contracts with a half dozen health systems—far exceeding our original projections.
The NeuroBlu Experience
At Holmusk, I witnessed a similar pattern with our flagship product, NeuroBlu Analytics. When I joined, the team was structured around the traditional Product Trio model, with data scientists consulted as needed but not integrated into core decision-making.
Early versions of the product faced challenges:
- Data models were technically sound but difficult for non-technical healthcare researchers to use
- Visualizations were beautiful but sometimes misrepresented statistical significance
- The platform excelled at showing correlations but offered little guidance on causation risks
As we evolved toward a Squad approach, with a dedicated Data Lead as a core team member, these issues began to resolve. The Data Lead became our ethical compass, constantly asking questions like:
- Are we providing enough context for these findings?
- Could this visualization lead researchers to draw inappropriate conclusions?
- Are we properly communicating the limitations of real-world evidence?
This shift accelerated our platform's adoption among life science companies—groups that need a ton of support to over come general skepticism of our commercial real-world evidence approach. They recognized and respected the ethical rigor our Data Lead brought to the product.
Building Your Own Data Product Squad
If you're developing a data product, how do you implement the Squad approach?
1. Elevate data expertise to leadership level
The Data Lead isn't just a technical contributor—they need authority equal to the other leaders. They should be present for strategic decisions from day one, not consulted afterward.
2. Look for T-shaped data expertise
The ideal Data Lead has depth in one area (e.g., data science, data engineering, data visualization or data governance) but breadth across the entire data lifecycle. They should understand enough about each area to identify risks and ask the right questions.
3. Value domain knowledge
Domain expertise is particularly critical for the Data Lead. In healthcare, for instance, understanding HIPAA, clinical workflows, and healthcare economics is as important as technical skills.
4. Create clear decision rights
Define which team member has final say in which areas. The Data Lead should have veto power on issues of data quality, model performance, and ethical use.
5. Establish data ethics principles
Work as a Squad to define ethical boundaries before you're faced with difficult tradeoffs. Document these principles and review them regularly.
The Future of Data Product Teams
Marty Cagan recently published a thought-provoking vision for how AI might reshape product teams, predicting that "product discovery will become the main activity of product teams, and gen ai-based tools will automate most of the delivery."
But even in this AI-accelerated future, Cagan still sees the need for specialized roles: "product teams will need a product manager to solve for the many business constraints, a product designer to solve for the user experience, and an engineer to solve for the technology."
For data products, I'd argue the same logic applies to the Data Lead. As AI becomes more integrated into products of all types, the need for data expertise at the leadership level will only grow, not diminish.
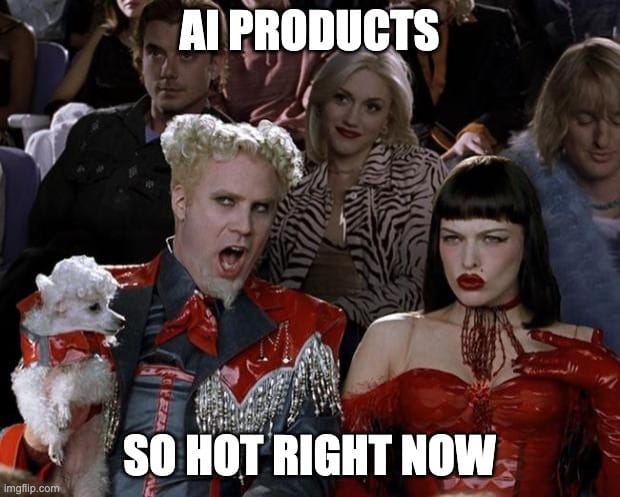
The line between "regular products" and "data products" will continue to blur. Eventually, all digital products may need something like the Squad approach.
But for now, if you're explicitly building a data product—particularly one that uses machine learning, predictive analytics, or works with sensitive information—the traditional Product Trio isn't enough.
You need a Data Product Squad, with the Data Lead as an essential fourth pillar.
Because data products don't just carry technical and market risks—they carry ethical risks too. But at the end of the day - not much is different. You still need to figure out what and how to build and distribute something that users value.
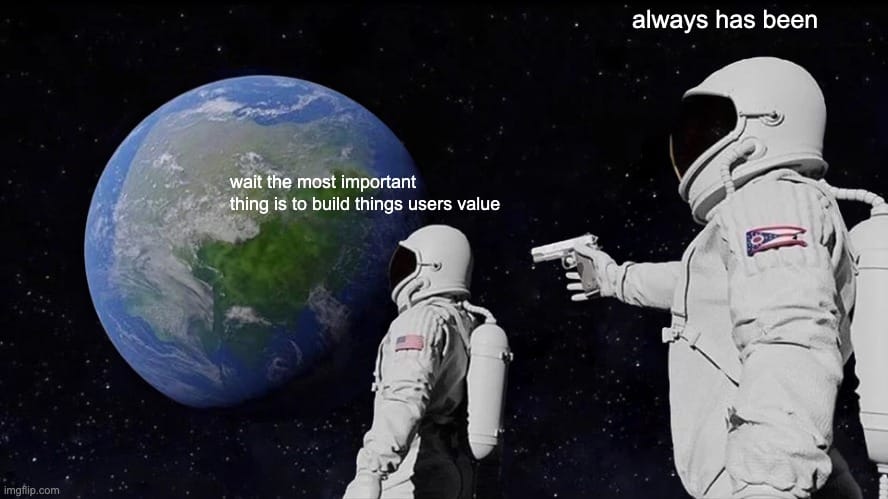
The Questions Nobody's Asking
- How do we measure the impact of ethical data product decisions on long-term customer trust?
- What skills and training do Product Managers need to work effectively with Data Leads?
- How does the Squad approach scale across multiple product teams in larger organizations?
- In an AI-augmented future, will the Data Lead become even more critical as ethical risks multiply?
- How do we balance innovation speed with ethical risk management in data product teams?
Would love to hear your thoughts. Have you seen the need for a dedicated Data Lead on your data product teams? What challenges have you faced when developing data products with traditional team structures?